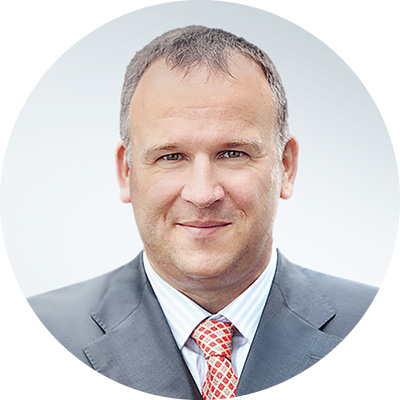
AUTOMOTIVE INDUSTRY LEVERAGING GenAI AND LARGE LANGUAGE MODELS (LLMs)
VP Europe, Grid Dynamics
Hybrid Search
Grid Dynamics has extensive experience in data-intensive systems, stemming from its early work with space-based architectures and technologies such as GigaSpaces, and later with ElasticSearch clusters powering faceted search on high-traffic websites. As search experts, we are now witnessing an evolution of these secondary databases. The traditional approach of lexical techniques is being expanded to handle embeddings or vector representations of language that can be understood by LLMs. This new representation has led to new types of databases that support the semantic algorithms for search. The combination of lexical and semantic techniques provides hybrid search capabilities, enabling use cases such as Retrieval-Augmented Generation (RAG) [6].
Language-based Assistants
In addition to hybrid search, the second capability that can extend digital solutions is the use of language-based assistants. This capability further expands the boundaries of what can be delegated to language models, and what aspects should be handled by traditional orchestration of services composed to achieve a particular goal. We see this at the very center of the language-based assistant stack.
Orchestration is essential as teams explore which generalization tasks can be delegated to a particular LLM, and which tasks require bespoke solutions. Two orchestration flows gaining traction are »Chain of Thought« and »ReAct«.
LLMOps
As with other data-intensive solutions in the applied machine learning space, platform aspects and the ability to sustain a delivery cadence with robust DORA metrics are essential. Grid Dynamics has outlined the functional and technical designs of an LLM platform in its blog, LLMOps blueprint for closed-source large language models [7]. We have specifically examined closed-source LLMs, and provided mappings to well-established and emerging frameworks, libraries, and products that can be used for implementation.
The ability to interact with data-intensive applications using natural language is a natural progression for modernizing many systems that were developed one or two decades ago. At Grid Dynamics, we have evolved from data-intensive systems for power users to wizards designed with UX concerns in mind. Implementing robust LLMOps practices, which encompass the processes, tools, and best practices for managing the entire life-cycle of LLM-powered applications, is crucial for organizations seeking to leverage these powerful models effectively and sustainably.
[1] Four ways conversational AI transforms digital experiences
[2] Insights by Grid Dynamics
[3] Transform your product design processes and personalization services with generative AI
[4] From design to delivery: The role of artificial intelligence in the automotive industry
[5] Why Unstructured Data Is Your Organization’s Best-Kept Secret – GeekWire
[6] RAG and LLM business process automation: A technical strategy – Grid Dynamics
[7] LLMOps blueprint for closed-source large language models
SUPPORT LINKS
Mercedes-Benz Elevates Production with ChatGPT – The GenAI Gazette
[2304.14721] Towards autonomous system: flexible modular production system enhanced with large language model agents
[2310.09536] CarExpert: Leveraging Large Language Models for In-Car Conversational Question Answering